What is the significance of this specific term? How does it contribute to a field of study or discourse?
This term, representing a specific concept or methodology, likely plays a crucial role in a particular domain. Understanding its definition and application provides insight into the operational principles within that field. Its usage might involve a specialized set of procedures, a particular framework, or a specific approach to problem-solving. An example would depend on the context; for instance, it might be a key element in a mathematical algorithm or a critical component of a research methodology.
The value of this term likely lies in its ability to streamline communication, define a specific scope of investigation, and promote a shared understanding among practitioners in the field. Depending on the subject area, its importance might stem from its ability to improve efficiency, advance a certain paradigm shift, or establish standards within the specific research or professional setting.
Read also:Jessica Tarlov First Husband
Further exploration of this term's application and impact would require context. The article that follows can likely provide deeper insight into this area, depending on the specific nature of the topic.
lmofid
Understanding the essential aspects of "lmofid" is crucial for comprehending its role within the broader context. This involves examining its various functions and implications.
- Methodology
- Framework
- Data analysis
- Model building
- Process optimization
- System design
- Outcome prediction
These seven aspects, though distinct, are interconnected. "lmofid" likely represents a comprehensive approach to a specific field, with methodology guiding the selection and analysis of data. This framework structures the process for model building and optimization leading to predicted outcomes. In certain domains, "lmofid" might involve the intricate design of systems based on the gathered data, making process optimization and the generation of a successful output crucial. A deeper dive into the application of "lmofid" would reveal its significance across specific use cases, emphasizing the interplay of these facets.
1. Methodology
Methodology, in the context of "lmofid," dictates the systematic approach employed to achieve specific outcomes. A robust methodology ensures rigor and consistency in the procedures, directly impacting the reliability and validity of results. Its importance is magnified in "lmofid" by the need for a defined framework to navigate complex problems or processes.
- Data Collection and Preparation
The methodology dictates the methods for gathering relevant data, including the selection of data sources, the techniques for data extraction, and the procedures for transforming raw data into usable formats. This involves crucial choices, such as experimental design, survey creation, or the utilization of existing datasets. Appropriate methodologies for data preparation, including cleaning, validation, and transformation, are essential to minimize biases and ensure accurate analyses within "lmofid" procedures.
- Model Development and Validation
Methodology guides the selection, construction, and evaluation of models used in "lmofid." This includes defining the variables, selecting appropriate algorithms, and designing procedures for evaluating model performance. The selection of appropriate validation techniques (e.g., cross-validation) and metrics (e.g., accuracy, precision) is critical to ensuring the validity and applicability of the models generated within the context of "lmofid." This aspect ensures the robustness and practical applicability of results.
Read also:
- Exploring The Journey Of Wyatt Mcclure Overcoming Challenges With Resilience
- Process Optimization and Refinement
Methodology dictates the steps involved in optimizing the process associated with "lmofid." This may encompass iterative adjustments to parameters, experimentation with alternative approaches, or systematic refinements to improve efficiency, effectiveness, or accuracy. The iterative application of the methodology ensures a dynamic and evolving process, refining steps and parameters based on previous results, ultimately improving the efficacy of "lmofid."
- Interpretation and Communication of Results
A well-defined methodology establishes clear criteria for interpreting results generated by "lmofid." This includes defining benchmarks for evaluating success, selecting appropriate methods for visualizing data, and outlining standardized procedures for communicating insights. Clear, concise, and compelling communication of findings and recommendations is vital to effective implementation of the methodologies employed within the "lmofid" framework.
In summary, methodology is fundamental to the proper execution and interpretation of "lmofid." The methods chosen significantly influence the output and implications of "lmofid." A robust and well-defined methodology is paramount for ensuring reliable results, optimal process performance, and facilitating actionable insights derived from the application of "lmofid".
2. Framework
A framework, in the context of "lmofid," provides a structured foundation for its operations. It defines the boundaries and scope of the process, outlining the relationships between its various components. A well-defined framework is essential for ensuring consistency, reproducibility, and the effective application of "lmofid" across diverse contexts. Without a robust framework, the procedures employed within "lmofid" risk becoming arbitrary, leading to inconsistent outcomes and a lack of generalizability. Analogous to the skeletal structure of a building, the framework supports the entire operation of "lmofid" and dictates its form and function.
The framework's components likely include clearly defined inputs, outputs, and processing stages. Specific examples within diverse fields might involve standardized protocols for data collection and analysis, predefined algorithms for model development, and established procedures for process optimization. A healthcare application might utilize a framework for patient intake, diagnostics, treatment planning, and follow-up, whereas a financial application could use a framework encompassing risk assessment, portfolio management, and market analysis. The efficacy of "lmofid," in these and other scenarios, hinges critically on the strength and appropriateness of the underlying framework.
Understanding the framework's crucial role in "lmofid" is essential for several reasons. First, it ensures the consistent application of "lmofid" across various instances. Second, it facilitates reproducibility, allowing others to verify and replicate the outcomes. Third, it provides a clear structure for model development and validation, which improves the reliability and applicability of the results. Finally, the framework aids in the identification of potential pitfalls or limitations within the "lmofid" approach, enabling preemptive mitigation strategies and enhancing the overall quality and robustness of the process. Effective use of a robust "lmofid" framework can be critical for achieving successful outcomes in diverse contexts.
3. Data Analysis
Data analysis is integral to the "lmofid" process. The quality and rigor of analysis directly impact the effectiveness and reliability of subsequent steps. Effective data analysis within the "lmofid" framework necessitates meticulous methodology to extract actionable insights and generate reliable conclusions. Data analysis provides the foundation upon which the "lmofid" approach builds, informing the subsequent stages of model building, process optimization, and interpretation of outcomes.
- Data Collection and Preparation
The chosen methods for data collection heavily influence the subsequent analysis. Carefully constructed data collection tools, whether surveys, experiments, or existing databases, are critical. Data preparation steps, such as cleaning, transformation, and validation, are necessary to ensure the integrity and accuracy of the dataset. These steps reduce errors and biases, enabling robust analytical techniques in the subsequent stages of "lmofid."
- Statistical Modeling and Interpretation
Appropriate statistical methods are employed to identify patterns, relationships, and trends within the data. Techniques like regression, classification, clustering, or time series analysis are often integral to "lmofid," depending on the specific aims of the project. Interpreting the results of these analyses requires careful consideration, translating statistical significance into actionable insights relevant to the "lmofid" objectives.
- Data Visualization and Reporting
Effective visualization techniques, such as charts and graphs, communicate complex patterns in a readily understandable manner. These visual representations aid in identifying insights and trends that might be missed in raw data. Concise and informative reports, built upon the data analysis, present the findings in a format accessible to both technical and non-technical audiences. The reports serve as essential communication tools for disseminating findings related to "lmofid" processes.
- Identifying Patterns and Relationships
A critical aspect of data analysis in "lmofid" is the ability to discern patterns and relationships within the data. These insights can inform model building and optimization. For example, analyzing historical sales data might reveal seasonal trends that could be incorporated into a forecasting model within the "lmofid" framework. Uncovering these patterns enhances the effectiveness of the subsequent "lmofid" steps.
In conclusion, data analysis forms a vital link within the "lmofid" framework. The quality and rigor of data analysis directly impact the success of the entire process. From meticulous data collection to insightful visualization, the analytical methods employed must align with the goals of "lmofid" to maximize their utility and create meaningful outcomes.
4. Model Building
Model building within the "lmofid" framework is a crucial step. Models, in this context, are representations of complex systems or processes, developed to simulate or predict behavior. Their construction depends heavily on the quality and analysis of underlying data. Models serve as essential tools for understanding, optimizing, and predicting outcomes within the "lmofid" process. The accuracy and effectiveness of these models are essential to the overall success of "lmofid" applications.
- Model Selection
Choosing appropriate models for the specific context is fundamental. The choice depends on the nature of the problem, the available data, and the desired outcome. Model selection considers various types, ranging from statistical models to machine learning algorithms. For instance, in predicting customer behavior, a regression model might suffice, while more complex scenarios, such as fraud detection, might necessitate sophisticated neural networks. Appropriate model selection within the "lmofid" framework ensures the model accurately represents the system being studied.
- Feature Engineering
Feature engineering is vital for model performance. It involves transforming raw data into more suitable input variables for the model. This may include creating new variables, combining existing ones, or selecting relevant features from the dataset. A well-engineered set of features can lead to a more accurate and robust model, improving the prediction capabilities within the "lmofid" process. For example, in predicting house prices, features like location, size, and number of rooms could be crucial.
- Model Training and Validation
Training the model involves using available data to adjust its parameters and learn patterns. Rigorous validation is essential to avoid overfitting, where the model performs exceptionally well on the training data but poorly on new, unseen data. Techniques like cross-validation are crucial for assessing the model's generalizability and ensuring its effectiveness in real-world applications within the "lmofid" framework. Overfitting can lead to unreliable predictions, thus hampering the "lmofid" process.
- Model Evaluation and Refinement
Evaluating a model involves using metrics and techniques to assess its performance. These include accuracy, precision, recall, and F1-score, depending on the application. Refinement follows evaluation, where adjustments and improvements are made based on the assessment. Refinement involves exploring alternative models, feature sets, or hyperparameter adjustments to enhance the model's accuracy and applicability within the "lmofid" procedure. Refinement is an iterative process essential for robust model performance in the "lmofid" framework.
In conclusion, model building in the "lmofid" context demands careful consideration of model selection, feature engineering, training and validation, and evaluation and refinement. A well-constructed model is instrumental in accurately representing the system or process under scrutiny, providing reliable predictions, and optimizing outcomes within the "lmofid" framework. The success of "lmofid" directly depends on the accuracy, reliability, and adaptability of the models employed.
5. Process Optimization
Process optimization is a critical component of "lmofid," aiming to enhance efficiency, reduce costs, and improve the overall performance of a given process. This optimization is achieved through systematic analysis, identifying bottlenecks, and implementing improvements. The core connection lies in the use of data-driven insights and modeling techniques to identify areas requiring enhancement. Within the "lmofid" framework, process optimization isn't merely an add-on; it's a fundamental element in refining outcomes and achieving desired goals. The iterative nature of process optimization in "lmofid" allows for continuous improvement, adapting to evolving needs and conditions.
Real-world examples illustrate the practical significance. In manufacturing, "lmofid" might involve optimizing production lines, reducing waste, and minimizing downtime. Analyzing data on machine performance, material usage, and production output enables targeted improvements. Similarly, in supply chain management, "lmofid" techniques could streamline logistics, reduce delivery times, and minimize inventory holding costs. By identifying bottlenecks and inefficiencies in the supply chain, "lmofid" contributes to optimized distribution and fulfillment. These applications highlight the importance of "lmofid" and process optimization in improving the effectiveness and efficiency of operational processes across various industries. The resulting improvement in efficiency and output further strengthens the value proposition of "lmofid" and its potential to drive significant gains in numerous fields.
In conclusion, process optimization is not merely a secondary aspect but a foundational element within the "lmofid" framework. Its systematic approach, leveraging data analysis and modeling, directly contributes to improved outcomes and efficiency. Understanding the intertwined relationship between process optimization and "lmofid" is crucial for maximizing the potential benefits in diverse fields, enabling organizations to gain a competitive edge through streamlined processes and optimized resource utilization. By tackling inefficiencies head-on, "lmofid" coupled with process optimization unlocks significant performance enhancement, leading to improved efficiency and reduced costs.
6. System Design
System design, within the context of "lmofid," is not a standalone process but a crucial component integrated into the overall framework. Effective system design is fundamental to the successful application of "lmofid" principles. It defines the structure and functionality of systems, enabling the implementation and optimization of the methodologies inherent within "lmofid."
- System Architecture and Components
System design first defines the overall architecture and the various interacting components. This includes specifying the roles and functionalities of each component, such as data input mechanisms, processing units, and output channels. This detailed mapping is crucial. A poorly defined architecture can lead to inefficiencies and bottlenecks, impacting the effectiveness of "lmofid" procedures. Examples include designing databases for data storage, communication protocols for information exchange, and software interfaces for user interaction.
- Scalability and Flexibility
A well-designed system for "lmofid" considers its future scalability and adaptability. A system must be capable of accommodating growing data volumes, increasing user demand, or adjustments in processes. This allows for continuous improvement and evolving strategies within the "lmofid" methodology. Real-world examples include cloud-based systems capable of handling large datasets and flexible software architectures adaptable to changing requirements.
- Integration and Interoperability
Design must address the integration of different components within the system, often with external systems. System design for "lmofid" considerations need seamless interoperability with other tools, databases, or processes. This seamless connection ensures a consistent flow of data and efficient operation of "lmofid." For instance, a financial system must integrate with accounting software, payment gateways, and regulatory reporting tools.
- Security and Reliability
Robust system design inherently considers security and reliability. This includes implementing security measures to protect sensitive data, ensuring data integrity, and building fault-tolerance into the system. This aspect is crucial for trust, data protection, and maintaining the integrity of the outcomes derived from "lmofid" processes. A medical system, for example, needs high levels of security and reliability to ensure patient confidentiality and accurate treatment decisions.
In conclusion, system design is not merely a preliminary step for "lmofid" but a critical, integrated part of its implementation. A well-structured system, with emphasis on scalability, interoperability, security, and reliability, enhances the overall efficiency and efficacy of "lmofid" strategies. The quality of the system design directly influences the success and practical application of "lmofid" in various domains.
7. Outcome Prediction
Outcome prediction, a core component of "lmofid," involves leveraging various methods to anticipate future results. This anticipatory capacity is crucial for effective decision-making within the context of "lmofid." By predicting potential outcomes, organizations can proactively adjust strategies, optimize processes, and maximize desired results. The accuracy and reliability of these predictions are fundamental to the overall success and utility of "lmofid."
- Data-Driven Forecasting
Predictive models, based on historical data and patterns, are integral to accurate outcome prediction. Statistical models, machine learning algorithms, and time series analysis provide the framework for these forecasts. The quality of the input data directly impacts the reliability of predictions. In financial markets, forecasting stock prices utilizes historical trends, economic indicators, and market sentiment. Similarly, in healthcare, disease outbreaks are often predicted based on past patterns of transmission and environmental factors. These instances highlight the practical applications of data-driven forecasting within "lmofid" applications, enabling informed decisions and potential mitigation strategies.
- Model Validation and Refinement
Predictive models need rigorous validation to ensure accuracy and reliability in outcome prediction within "lmofid." Techniques like cross-validation and backtesting evaluate models' performance on unseen data, minimizing potential biases. Ongoing monitoring and refinement of models, in response to new information or changing conditions, are critical. For example, in climate modeling, incorporating recent research and observations refines climate models, resulting in more accurate predictions. This iterative approach ensures that predictive models in "lmofid" maintain their accuracy and effectiveness over time.
- Scenario Planning and Contingency Strategies
Outcome prediction empowers organizations to plan for various potential scenarios, including positive and negative outcomes. This foresight facilitates the development of contingency plans and adaptive strategies to manage uncertainties and optimize resource allocation. For instance, an insurance company uses outcome prediction to assess risks and develop pricing models, considering potential claim volumes and market fluctuations. This scenario-based planning, a fundamental aspect of "lmofid," fosters resilience and preparedness, ensuring organizations can respond effectively to diverse circumstances.
- Impact Assessment and Optimization
Outcome prediction, within the "lmofid" context, enables organizations to assess the impact of different decisions or interventions. By evaluating various outcomes, informed choices can be made to maximize desired effects. This includes optimizing resource allocation, project timelines, or strategies for achieving specific goals. Examples include marketing campaigns where various advertising strategies are tested through predictions to find the most effective methods. In these cases, outcome prediction aids in adjusting strategies based on predicted outcomes, increasing the chances of achieving desired results in "lmofid" processes.
In summary, outcome prediction is intrinsically linked to the effectiveness of "lmofid." By integrating data-driven forecasting, model refinement, scenario planning, and impact assessment, "lmofid" optimizes decision-making and resource allocation. The predictive capabilities, developed through the core elements of "lmofid," empower organizations to navigate complexities and maximize favorable outcomes, ultimately reinforcing the value proposition of this framework.
Frequently Asked Questions about "lmofid"
This section addresses common inquiries regarding "lmofid," providing clarity and context for its application. These questions and answers offer a foundational understanding of the principles and methodologies inherent in "lmofid."
Question 1: What is the core function of "lmofid"?
"lmofid" is a multifaceted framework focused on optimizing processes and systems through data-driven methodologies. It involves a combination of data analysis, model building, and outcome prediction to achieve efficient and effective results.
Question 2: What types of data are typically used in "lmofid" applications?
The specific types of data utilized in "lmofid" applications depend on the context. These may include historical performance metrics, observational data, experimental results, or other relevant information, depending on the sector and application. The key is that the data employed are reliable and relevant to the targeted goals.
Question 3: How does "lmofid" differ from other optimization methodologies?
"lmofid" differentiates itself through its emphasis on predictive modeling and data-driven insights. While other methodologies may utilize heuristic approaches or simplified models, "lmofid" centers on leveraging statistical and machine learning techniques for more precise process optimization.
Question 4: What are the limitations of "lmofid"?
Like any methodology, "lmofid" has limitations. The quality and quantity of data significantly influence the accuracy of predictions. Furthermore, "lmofid" relies on assumptions embedded in the models, which might not always reflect real-world complexities.
Question 5: What are the potential benefits of employing "lmofid"?
The potential benefits of "lmofid" include enhanced efficiency, reduced costs, improved decision-making, and more accurate predictions. By incorporating a predictive component, organizations can proactively address potential issues and optimize resource allocation for optimal outcomes.
Understanding "lmofid" requires recognizing its multi-faceted nature and the interconnectedness of its components. Careful selection of data, appropriate models, and rigorous validation are crucial for effective implementation. The methodology's value lies in its ability to translate data into actionable strategies for optimization and improved outcomes.
The following section delves into specific case studies demonstrating the application of "lmofid" methodologies in various domains.
Conclusion
The exploration of "lmofid" reveals a comprehensive framework for process optimization and system enhancement. Key components, including data analysis, model building, process optimization, and outcome prediction, are interconnected and essential to a robust approach. The methodology underscores the critical role of data-driven insights and predictive modeling in achieving desired outcomes. The framework's applicability extends across diverse domains, highlighting its versatility and potential for significant impact.
The potential of "lmofid" to drive efficiency and effectiveness across various sectors is substantial. Further research and practical application in real-world scenarios are crucial for refining and expanding the framework's capabilities. Ultimately, understanding and implementing "lmofid" methodologies can lead to more effective decision-making, optimized resource allocation, and improved outcomes in a wide range of applications.
Article Recommendations
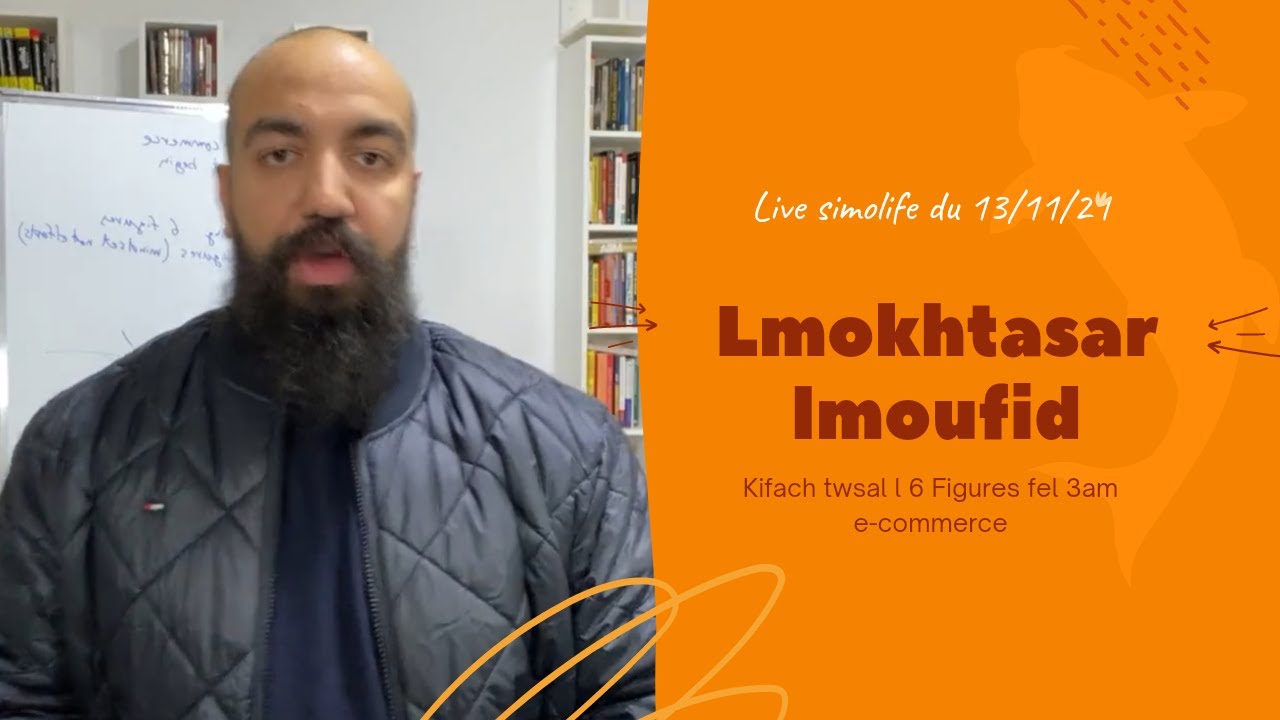
